I wrote one of the fastest DataFrame libraries
February 28, 2021
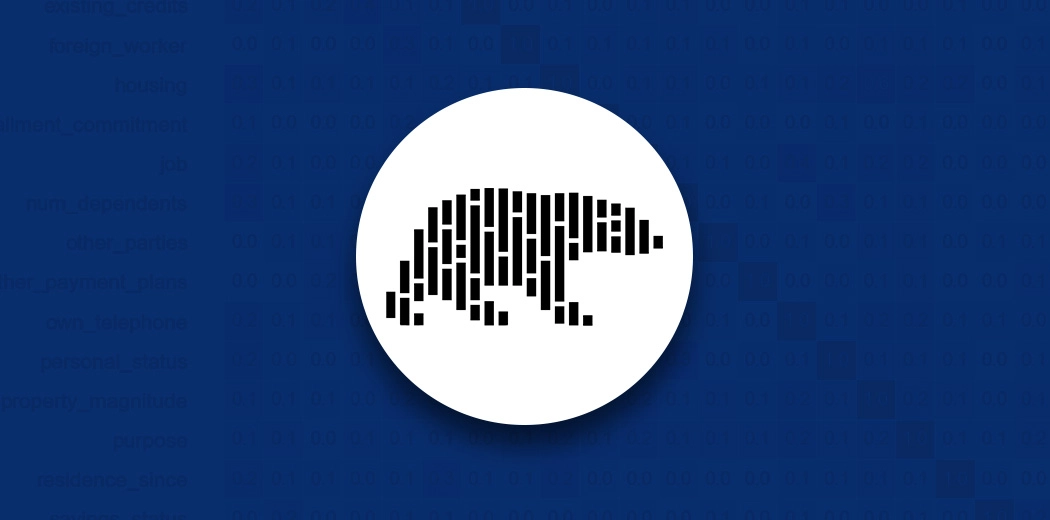
1. Introduction At the time of writing this, the coronavirus has been in our country for a year, which means I have been sitting at home for a very long time. At the start of the pandemic, I had a few pet projects in Rust under my belt and I noticed that the “are we DataFrame yet”, wasn’t anywhere near my satisfaction. So I wondered if I could make a minimalistic crate that solved a specific use case of mine.
Read more